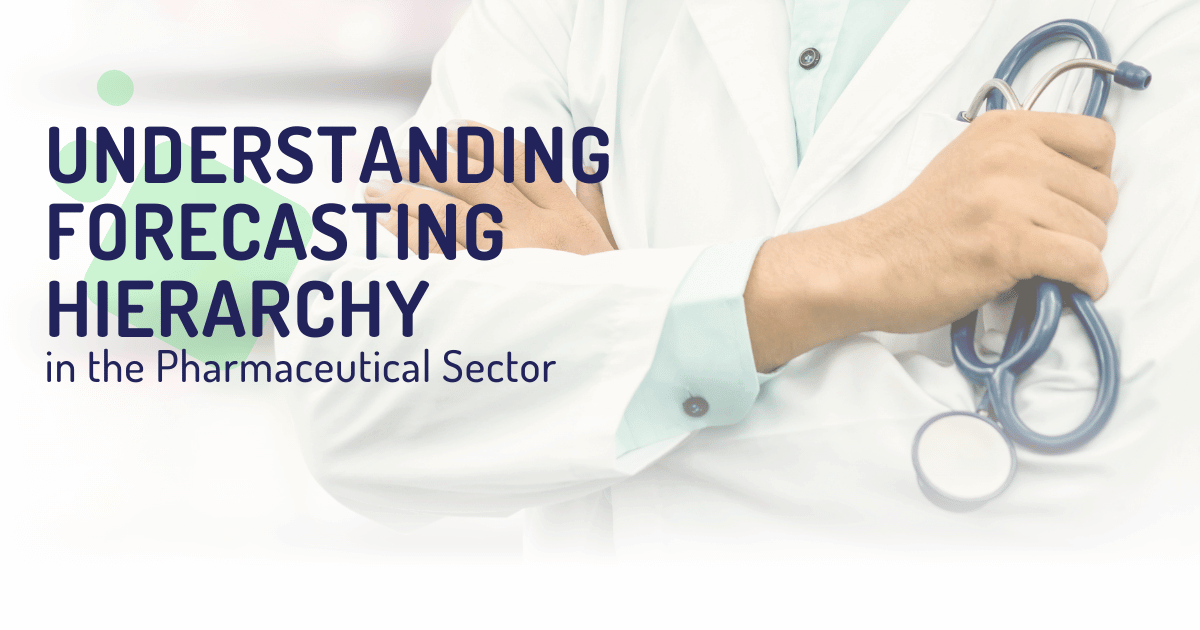
In the pharmaceutical sector, one of the most critical topics is forecasting, a fundamental activity for production planning and supply chain optimization. Forecasting is central because the actual availability of drugs in the market directly depends on its accuracy, influencing the company’s ability to meet demand and set strategic objectives.
A crucial element of this process is the forecasting hierarchy, which allows data to be broken down into various levels of aggregation. This methodology, also known as the forecasting hierarchy, enables data analysis through a tree structure, where each level represents a different degree of temporal, geographical, or product aggregation. In this context, each level offers a different level of detail, providing both granular and comprehensive views of forecasts.
In the pharmaceutical sector, the forecasting hierarchy helps manage the complexity of data from various sources, such as supply chain, sales, and market research. This method not only facilitates collaboration between country managers, regional leaders, and account managers but also allows for cumulative forecast values to be visualized at the controlling account level, improving transparency and decision-making efficiency.
By using machine learning techniques and advanced analytics tools, companies can manage and analyze large amounts of historical and scenario data, simulating various market events to enhance the accuracy of their forecasts while reducing uncertainty and optimizing resources.
This approach considers variables such as the product hierarchy, patient population growth, research innovations, emerging competing products, and government policies, ensuring more accurate and reliable forecasts. The adoption of series forecasting models within a hierarchical structure also allows for future scenario simulations and timely reactions to market changes, thereby improving decision-making and competitiveness in the pharmaceutical industry.
The Three Dimensions of Forecasting
Demand forecasting in the pharmaceutical sector is based on three main dimensions: materiality, geography, and temporality. These dimensions form the foundation of the forecasting hierarchy and provide a detailed and precise view of future demand.
- Materiality: Forecasts can be made at different levels of detail, such as SKU, product, segment, brand, etc. They can also be measured with various metrics, including units, value, and active ingredients.
- Geography: Demand can be forecasted based on various geographical criteria, such as country, region, market, channel, customer segment, or warehouse.
- Temporality: Forecasts can be made across different time horizons, ranging from daily to weekly, monthly, quarterly, or annually.
In addition to these three main dimensions, another crucial aspect is the forecasting horizon, which indicates how many periods ahead need to be forecasted. This can vary significantly depending on business needs, ranging from one week to two years or more. In the pharmaceutical sector, the forecasting horizon is particularly important for supply chain management and resource planning, as it allows for quick adaptation to market changes and research innovations.
Scaling Forecasts Up or Down
The forecasting hierarchy is a crucial element in the pharmaceutical sector, where forecast accuracy can significantly impact the supply chain and product availability. Forecasts can be generated at various levels of aggregation, such as store, day, and product, or month, country, and product family.
To optimize this process, three main techniques can be used:
- bottom-up: Aggregated forecasts are created by summing the granular levels downstream. For example, summing the forecasts of each warehouse in a country to obtain a national-level forecast.
- top-down: Detailed forecasts are created by disaggregating high-level forecasts using simple rules or historical weighted averages. For instance, a monthly forecast can be broken down into daily forecasts using a flat distribution or based on historical retail revenue.
- middle-out: This intermediate technique uses a single forecast to generate both a more aggregated view and a detailed version. For example, starting with a country-level forecast, it can be summed up by geographical region and distributed by retail point.
The adoption of hierarchical forecasting models in the pharmaceutical sector not only improves forecast accuracy but also allows for more efficient resource management and better responsiveness to market dynamics. By leveraging machine learning algorithms and advanced platforms (such as Profiter’s AI solutions for the pharmaceutical sector), companies can analyze series forecasting and simulate complex scenarios, ensuring greater responsiveness and adaptability.